Improve diagnostics of typhoid through Open Science: An Artificial Intelligence-based technique
- Track: Open Research Tools and Technologies devroom
- Room: D.research
- Day: Saturday
- Start: 13:45
- End: 14:05
- Video with Q&A: D.research
- Video only: D.research
- Chat: Join the conversation!
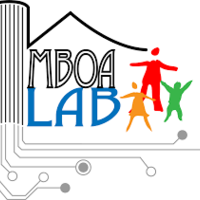
Typhoid fever is one of the severe infectious human diseases in Africa. Out of an estimated 11–21 million cases of typhoid fever and 200,000 deaths occur worldwide each year. Other elements contribute to this situation and particularly in rural areas where patients to doctors ratio is very low, lack of medical facilities and costly tests. There are a number of tests available presently, from molecular to immunological and biochemical to microbiological. However, Users are unsatisfied due to delays in getting test results and Imprecise diagnosis. Misdiagnosis is usually experienced since most health care facilities use only Widal test without confirmation of results with a second test method. In addition, the diagnosis of Typhoid involves several levels of uncertainties. Patients cannot tell exactly how they feel, doctors and nurses cannot tell exactly what they observe. There is therefore, an urgent need to develop a rapid, highly sensitive and cheap diagnostic tool for diagnosis of typhoid fever.
The absence of a reliable diagnosis pushes rural populations towards self-medication with all the consequences that this entails, in particular drug poisoning which can lead to death. As such, we want to develop a new method to diagnose Typhoid early, quickly, and with accurate results at a low cost. We propose a hybrid diagnosis method with a Deep Learning algorithm applied on blood serum images which seems to be the best accepted test by laboratory technicians. We use CNNs as algorithms on the collected images to train the algorithm. We use a second algorithm Rough Set Theory. This algorithm based on symptoms variables which are structured data collected from medical doctors allow us not only to confirm the diagnosis but above all to determine the level of severity of the disease and serve as a Decision Support System. The two algorithms will be part of a mobile app connected to an optical microscope, the phone camera will scan images from the slides on the microscope and classify them into Normal or Infected. In a series of rapid spotlights, we present tools and frameworks that serve as cornerstones for the envisioned diagnostics system including: 1) Values, influences and forces that shape the diagnostics 2) AI solutions to be used; 3) Data sources 4) Ethics and governance model.
Speakers
![]() |
Elisee JAFSIA |